Financial sector employees are being monitored: three examples from the sector
Automated payroll system, quality replacement based on data and AI analyses of employee conversations. Get insight into three cases from the sector revealing how employee data and AI are becoming increasingly important to companies.
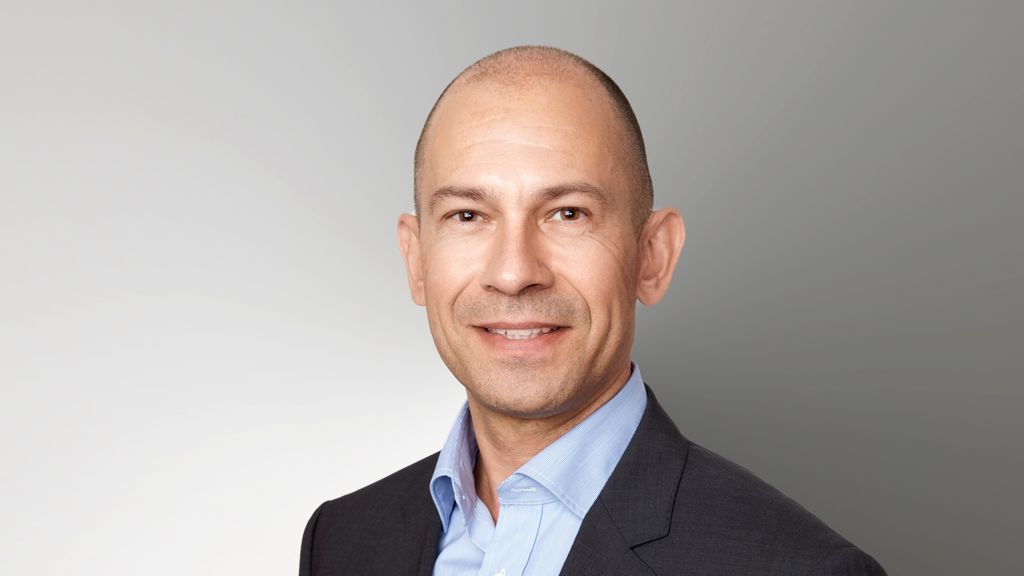
Case 1: Customer service and empathy score
In a customer service centre, phone calls are analysed using AI. The tone of voice, rate of speech and choice of words are assessed, and the employee is assigned an empathy score.
In addition, every conversation is evaluated based on up to 30 or 40 KPIs, such as correct presentation and closing remarks.
According to information obtained by Finansforbundet, it is the experience of several employees that they change their behaviour to achieve a high score – rather than to provide good service.
Systems and KPIs risk causing a changed behaviour among employees (so-called 'gaming' of the system), which is not necessarily productive in the long run.
"There are examples of employees beginning to adapt to the system to achieve good scores – rather than using their experience and skills to obtain results," says Johan Busse.
Johan Busse questions whether it is even possible to measure empathy, for example, in a phone call in this way.
"From a technical perspective, it looks very convincing, but the risk is using the technology for something it's not suited to."
"It may also cause what we call observational stress. When you're constantly listened to and evaluated by a system, it's like having a manager sit in on all your conversations. It affects the working environment, and it inhibits your freedom to apply your skills.
Case 2: Performance and "quality replacement"
In another organisation, front-office employees are assessed on their ability to handle cases effectively. Performance is, for example, measured by the number of completed cases, keystrokes, time spent on the phone and complexity of the case.
If the data does not indicate special circumstances to explain lower productivity, employees risk being laid off as part of a "quality replacement."
In general, it may cause a sense of injustice and insecurity if that type of data collection is not followed up by dialogue and contact with a manager who knows the particular employee.
"Data is never unbiased. It contains noise, bias and room for interpretation. If you don't know this, you risk making decisions that seem objective – but are actually deeply unfair," says Johan Busse and continues:
"A system may conclude that Henrik achieves a low score. But his manager knows that Henrik is essential to the team, because he helps new colleagues and fosters overall well-being.
Case 3: Using AI for salary reviews
A large organisation uses an AI tool to assist in the annual salary review. Based on an analysis of data from multiple sources – for instance courses, collaboration patterns and strategic importance – a prioritised list is compiled of candidates for a pay increase.
But to many employees, the system resembles a black box, where they have no idea of what is considered most important as they do not have access to the algorithm or its parameters.
According to Johan Busse, this is a break from Danish management traditions.
"We typically expect an explanation when we don’t receive a salary increase. Such explanation is lost when everything is based on an algorithm – and that may undermine the sense of fairness and create distrust," he says and goes on:
"In addition, the Danish Salaried Employees Act requires fairness and objectivity in connection with dismissals. The algorithm must meet these requirements."
According to Johan Busse, such system also risks favouring employees who achieve high scores in visible KPIs – but not necessarily those who contribute to the professional community in ways that are not measurable.
Trust requires transparency – and conversation
Johan Busse believes that the three cases are excellent examples of how the systems are not necessarily the problem. It is how we use them.
"This is a classic data ethics dilemma – while the systems themselves are not unethical, the way we use them may be. That's why openness is crucial, just as we need a forum to discuss it. The obvious place to go if you're worried or have any doubts are the union representatives and health and safety representatives," he says.
In 2024, the Danish Data Ethics Council published a 100-page analysis on data ethical perspectives regarding the use of employee data https://dataetiskraad.dk/dataetiske-temaer/dataetik-paa-arbejdspladsen. In the analysis, the Council explores the complex data ethical dilemmas that may arise from the collection and use of employee data in the workplace.
Earlier this year, the analysis was followed up by the free conversation game "Dataetik på Spil" ("data ethics at stake"), which may initiate important discussions about the use of data in the workplace.
"Data ethics is not about finding the right answer – but about asking the right questions. Trust and accountability emerge through dialogue between management and employees. It should be the workplace's data – not just the employer's."
"We tend to rely too much on data. But not everything that can be measured matters, and not everything that matters can be measured. We should therefore be cautious about allowing data systems to define our working lives without considering their impact on relations, management and culture."
Five data types typically collected about employees
- Access and location data
Data from access cards, Wi-Fi logging and booking of workstations shows when and where employees are located – primarily used for security, facility management and optimisation of office space. - Usage data from digital systems
Systems such as email, Teams, Slack, the intranet and calendars generate log data about communication, meeting activity and usage patterns. Often used for analysing workflows and collaboration. - Device and IT activity
Collection of keystrokes, file transfers, application usage and browser history. Primarily used for IT security and risk management. - Biometric access data
Fingerprint and facial recognition are increasingly used for physical and digital access control. - Voluntary and self-reported data
Data from well-being and employee surveys.
The greater the transparency, the greater the acceptance
Finansforbundet has for several years worked actively to put data ethics discussions on the agenda. We do this through political initiatives and practical tools – and by strengthening the role of union representatives in the technological issues increasingly shaping our working lives.
In 2023, Finansforbundet conducted an analysis revealing that, while many members have basic trust in their data being processed responsibly, only a few truly understand what data is used – and how.
"Greater knowledge of how AI and data are used leads to greater trust. Therefore, the Danish labour market's trust-based culture is crucial in our approach to AI," says Steen Lund Olsen, Vice President of Finansforbundet.
"It should be integrated into our collective collaboration, and that's why it's been agreed, in the new collective agreement, that the parties should discuss the use of employee data and together develop guidelines."
Finansforbundet generally recognises that new technologies such as AI and data-driven solutions are part of the business and working life of the financial sector.
“AI has great potential to improve our working environment and processes for the benefit of both the bottom line and the employees. It requires well-defined structures, employee involvement and awareness of how to leverage it. Because it's not just about what's possible and legal, but also about accountability” says Steen Lund Olsen
“Digital leadership must go hand in hand with ethical responsibility, integrity and trust.”
Ansvarlig AI
Ansvarlig AI kræver bl.a. medindflydelse, kompetenceudvikling og klare etiske retningslinjer.
Bliv klogere på ansvarlig AI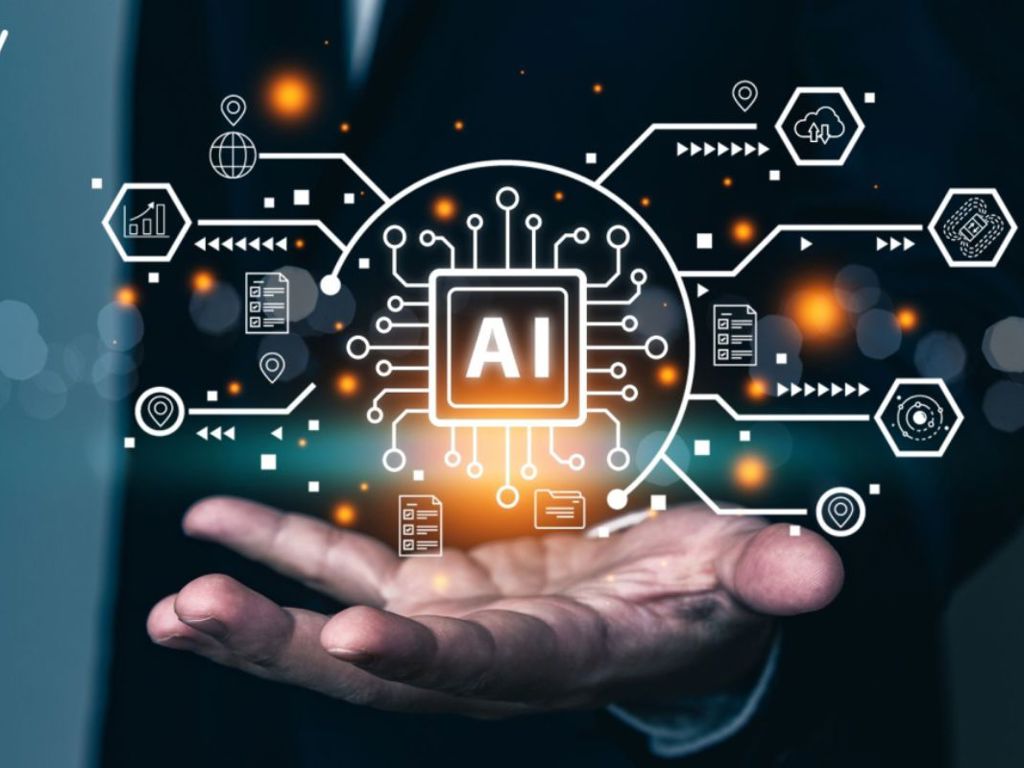